|
ACCESS THE FULL ARTICLE
No SPIE Account? Create one
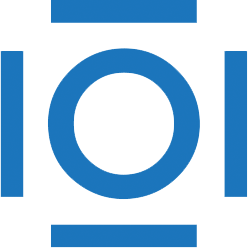
CITATIONS
Cited by 1 scholarly publication.
Denoising
Image classification
Medical imaging
Digital filtering
Electronic filtering
Performance modeling
Image denoising