|
ACCESS THE FULL ARTICLE
No SPIE Account? Create one
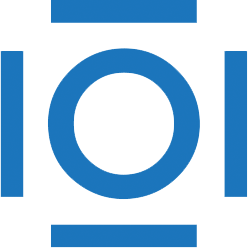
CITATIONS
Cited by 3 scholarly publications.
Signal to noise ratio
Imaging systems
Sensors
Target detection
Visual process modeling
Monte Carlo methods
Performance modeling