|
ACCESS THE FULL ARTICLE
No SPIE Account? Create one
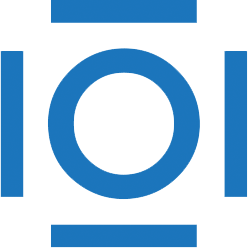
CITATIONS
Cited by 1 scholarly publication.
Image segmentation
Spine
3D modeling
Image resolution
Computed tomography
Image processing
Metals