|
ACCESS THE FULL ARTICLE
No SPIE Account? Create one
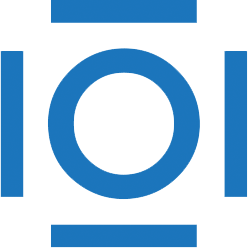
CITATIONS
Cited by 1 scholarly publication.
Feature extraction
Evolutionary algorithms
Convolution
Image classification
Buildings
Statistical modeling
Network architectures