Automatic and reliable stroke lesion segmentation from diffusion magnetic resonance imaging (MRI) is critical for patient care. Methods using neural networks have been developed, but the rate of false positives limits their use in clinical practice. A training strategy applied to three-dimensional deconvolutional neural networks for stroke lesion segmentation on diffusion MRI was proposed. Infarcts were segmented by experts on diffusion MRI for 929 patients. We divided each database as follows: 60% for a training set, 20% for validation, and 20% for testing. Our hypothesis was a two-phase hybrid learning scheme, in which the network was first trained with whole MRI (regular phase) and then, in a second phase (hybrid phase), alternately with whole MRI and patches. Patches were actively selected from the discrepancy between expert and model segmentation at the beginning of each batch. On the test population, the performances after the regular and hybrid phases were compared. A statistically significant Dice improvement with hybrid training compared with regular training was demonstrated (p < 0.01). The mean Dice reached 0.711 ± 0.199. False positives were reduced by almost 30% with hybrid training (p < 0.01). Our hybrid training strategy empowered deep neural networks for more accurate infarct segmentations on diffusion MRI. |
ACCESS THE FULL ARTICLE
No SPIE Account? Create one
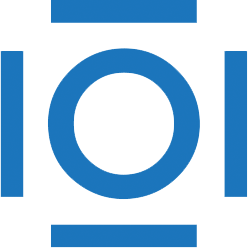
CITATIONS
Cited by 3 scholarly publications.
Magnetic resonance imaging
Image segmentation
Databases
Diffusion weighted imaging
3D modeling
Diffusion magnetic resonance imaging
Data modeling