Aiming at the problem of object blur in optical remote sensing images caused by atmospheric disturbance and cloud disturbance, a remote sensing image super-resolution reconstruction network, namely feature-enhanced reconstruction generative adversarial network (FR-GAN), based on a generative confrontation structure is proposed. The network adopts a self-supervised learning mode to achieve high-quality image reconstruction by learning the characteristics of the reconstructed image. First, to simulate the blurring effect of remote sensing images affected by interference factors, Gaussian noise is introduced into the image degradation model of self-supervised learning. Second, a background information enhancement module is designed in the FR-GAN image generator, which solves the problem of blurred objects in the reconstructed image by extracting the background contour information of the image and enhancing its expressive ability. Finally, the relative loss and perceptual loss are used to construct the loss function of FR-GAN. On the one hand, it improves the stability of the training process, and on the other hand, it improves the quality of image detail texture reconstruction. The experimental results show that, compared with the existing super-resolution reconstruction networks, the image reconstructed by FR-GAN has a clear texture, rich details, and better subjective visual effect. |
ACCESS THE FULL ARTICLE
No SPIE Account? Create one
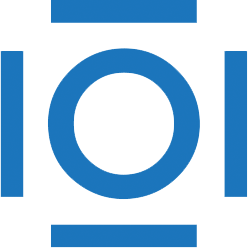
CITATIONS
Cited by 1 scholarly publication.
Remote sensing
Super resolution
Image processing
Convolution
Image restoration
Image quality
Lawrencium