To ease visual inspections of exterior aircraft fuselage, new technical approaches have been recently deployed. Automated unmanned aerial vehicles (UAVs) are now acquiring high-quality images of aircraft in order to perform offline analysis. At first, some acquisitions are annotated by human operators in order to provide a large dataset required to train machine learning methods, especially for critical defect detection. An intrinsic problem of this dataset is its extreme imbalance (i.e., there is an unequal distribution between classes). The rarest and most valuable samples represent few elements among thousands of annotated objects. Deep learning (DL)-only based approaches have proven to be very effective when a sufficient amount of data are available for each desired class, whereas few-shot learning (FSL)-dedicated methods (matching network, prototypical network, etc.) can learn from only few samples. In a previous work, those approaches were compared on our applicative case and it was demonstrated that combining DL model and prototypical neural network in a hybrid architecture improves the results. We extend this work by questioning the interface between models in such a hybrid architecture. We show that by carefully selecting the data from the well-represented class when using FSL techniques, it is possible to enhance the previously proposed solution. |
ACCESS THE FULL ARTICLE
No SPIE Account? Create one
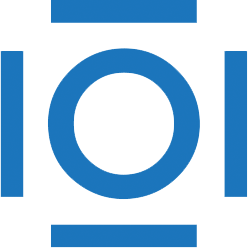
CITATIONS
Cited by 3 scholarly publications.
Data modeling
Neural networks
Prototyping
Image classification
Unmanned aerial vehicles
Machine learning
3D modeling