Modality-fused representation is an essential and challenging task in multimodal emotion analysis. Previous studies have already yielded remarkable achievements. However, there are two problems: insufficient feature interaction and rough data fusion. To investigate these two challenges more deeply, first, a hybrid architecture, which consists of convolution and a transformer, is proposed to extract local and global features. Second, for extracting more sufficient mutual features from multimodal datasets, our model is comprised of three parts: (1) the interior transformer encoder (TE) aims to extract the intramodality characteristics from the current monomodality; (2) the between TE aims to extract the intermodality feature between two different modalities; and (3) the enhance TE aims to extract the target modality enhance feature from multimodality. Finally, instead of directly fusing features by a linear function, we employ a popular and widely used multimodal factorized high-order pooling mechanism to obtain a more distinguishable feature representation. Extensive experiments on three multimodal sentiment datasets (CMU-MOSEI, CMU-MOSI, and IEMOCAP) demonstrate that our approach reaches the state-of-the-art in an unaligned version setting. Compared with the mainstream methods, our proposed method shows superiority in both word-aligned and unaligned settings. |
ACCESS THE FULL ARTICLE
No SPIE Account? Create one
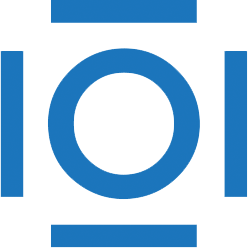
CITATIONS
Cited by 2 scholarly publications.
Transformers
Emotion
Data modeling
Video
Feature extraction
Convolution
Visualization