|
ACCESS THE FULL ARTICLE
No SPIE Account? Create one
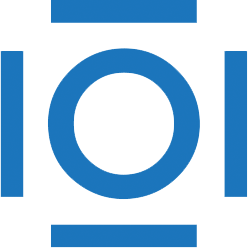
CITATIONS
Cited by 2 scholarly publications.
Image fusion
Associative arrays
Independent component analysis
Principal component analysis
Optical engineering
Medical imaging
Visualization