|
ACCESS THE FULL ARTICLE
No SPIE Account? Create one
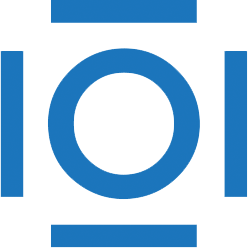
CITATIONS
Cited by 3 scholarly publications.
Lithography
Photomasks
Data modeling
Convolution
Data acquisition
Machine learning
Neural networks