|
ACCESS THE FULL ARTICLE
No SPIE Account? Create one
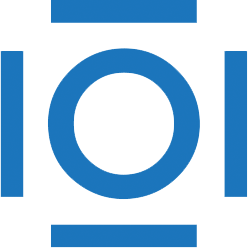
CITATIONS
Cited by 1 scholarly publication.
Minerals
Machine learning
Visualization
Statistical analysis
Data acquisition
Hyperspectral imaging
Sensors