|
ACCESS THE FULL ARTICLE
No SPIE Account? Create one
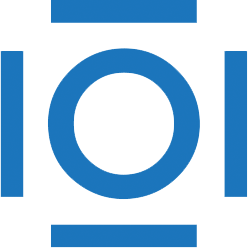
CITATIONS
Cited by 4 scholarly publications.
Inspection
Sensors
Cameras
Object recognition
Target detection
Detection and tracking algorithms
Neural networks