|
ACCESS THE FULL ARTICLE
No SPIE Account? Create one
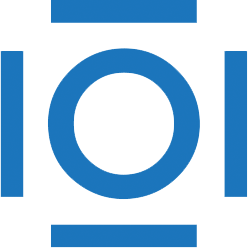
CITATIONS
Cited by 5 scholarly publications.
Unmanned aerial vehicles
Convolution
Detection and tracking algorithms
Remote sensing
Data modeling
Feature extraction
Image processing