|
ACCESS THE FULL ARTICLE
No SPIE Account? Create one
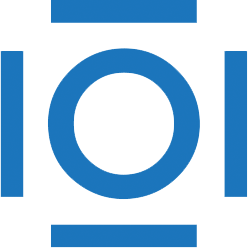
CITATIONS
Cited by 2 scholarly publications.
Computed tomography
Image restoration
Reconstruction algorithms
Image quality
Compressed sensing
Computer simulations
Medical diagnostics