|
ACCESS THE FULL ARTICLE
No SPIE Account? Create one
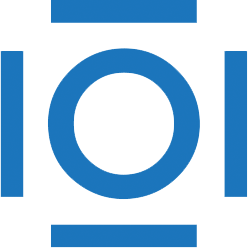
CITATIONS
Cited by 1 scholarly publication.
Image segmentation
Data modeling
Detection and tracking algorithms
Image resolution
Unmanned aerial vehicles
Airborne remote sensing
Binary data