|
ACCESS THE FULL ARTICLE
No SPIE Account? Create one
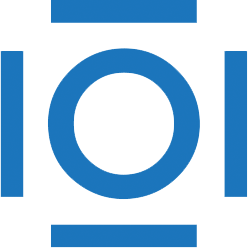
CITATIONS
Cited by 1 scholarly publication.
Convolutional neural networks
Convolution
Data modeling
Visualization