|
ACCESS THE FULL ARTICLE
No SPIE Account? Create one
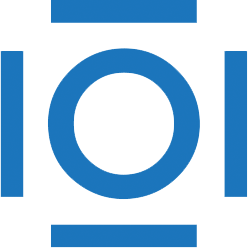
CITATIONS
Cited by 2 scholarly publications.
Neural networks
Neurons
Machine learning
Computer vision technology
Control systems
Machine vision
Robot vision