|
ACCESS THE FULL ARTICLE
No SPIE Account? Create one
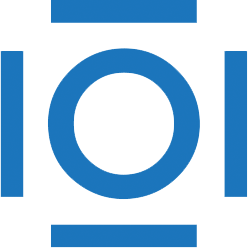
CITATIONS
Cited by 2 scholarly publications.
Binary data
Convolution
Neural networks
Feature extraction
Image processing
Databases
Image classification