|
ACCESS THE FULL ARTICLE
No SPIE Account? Create one
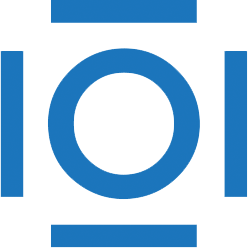
CITATIONS
Cited by 1 patent.
Neurons
Amplifiers
Capacitors
Chemical elements
Transistors
Artificial neural networks
Analog electronics