|
ACCESS THE FULL ARTICLE
No SPIE Account? Create one
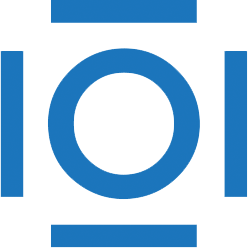
CITATIONS
Cited by 4 scholarly publications.
Sensors
Neural networks
Temperature sensors
Logic
Signal to noise ratio
Detector development
Data modeling