|
ACCESS THE FULL ARTICLE
No SPIE Account? Create one
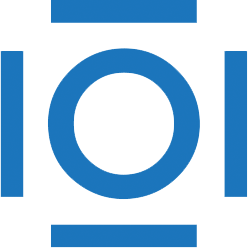
CITATIONS
Cited by 4 scholarly publications.
Neural networks
Neurons
Control systems
Complex systems
Nonlinear control
Data processing
Dynamical systems