|
ACCESS THE FULL ARTICLE
No SPIE Account? Create one
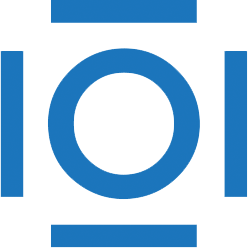
CITATIONS
Cited by 3 scholarly publications.
Principal component analysis
Silicon
Magnesium
Iron
Aluminum
Visualization
Calcium