|
ACCESS THE FULL ARTICLE
No SPIE Account? Create one
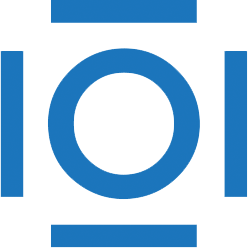
CITATIONS
Cited by 4 scholarly publications.
Image segmentation
Breast
Mammography
Tissues
Radiology
Statistical analysis
Cancer