|
ACCESS THE FULL ARTICLE
No SPIE Account? Create one
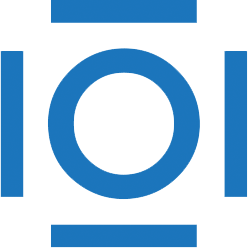
CITATIONS
Cited by 9 scholarly publications.
Sensors
Feature selection
Nondestructive evaluation
Feature extraction
Neural networks
Principal component analysis
Diagnostics