|
ACCESS THE FULL ARTICLE
No SPIE Account? Create one
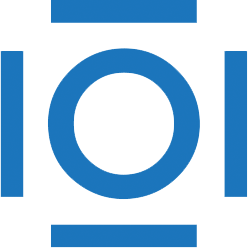
CITATIONS
Cited by 2 scholarly publications.
Neural networks
Thermal modeling
Infrared cameras
Thermography
Connectors
Data modeling
Artificial neural networks