|
ACCESS THE FULL ARTICLE
No SPIE Account? Create one
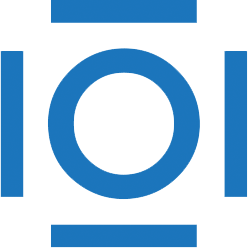
CITATIONS
Cited by 2 scholarly publications.
Tissues
Luminescence
Cancer
Breast cancer
Fluorescence spectroscopy
Light scattering
Statistical analysis