|
ACCESS THE FULL ARTICLE
No SPIE Account? Create one
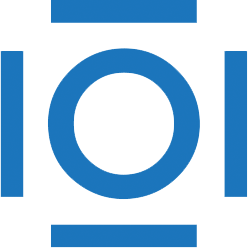
CITATIONS
Cited by 9 scholarly publications.
Image segmentation
RGB color model
Video surveillance
Image processing
Video
Data modeling
Computer vision technology