|
ACCESS THE FULL ARTICLE
No SPIE Account? Create one
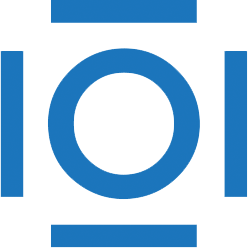
CITATIONS
Cited by 2 scholarly publications.
Image quality
Performance modeling
Medical imaging
Receivers
Feature extraction
Image filtering
Imaging systems