|
ACCESS THE FULL ARTICLE
No SPIE Account? Create one
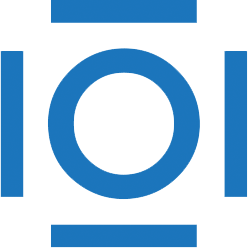
CITATIONS
Cited by 2 scholarly publications.
Stars
Astronomy
Spectroscopy
Performance modeling
Space telescopes
Statistical modeling
Telescopes