|
ACCESS THE FULL ARTICLE
No SPIE Account? Create one
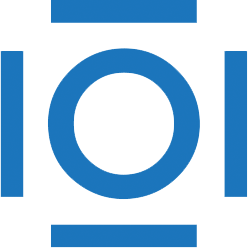
CITATIONS
Cited by 4 scholarly publications.
Image segmentation
Feature extraction
Image processing
Distortion
Image filtering
Mahalanobis distance
Detection and tracking algorithms