|
ACCESS THE FULL ARTICLE
No SPIE Account? Create one
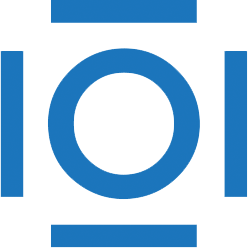
CITATIONS
Cited by 1 scholarly publication.
Composites
MODIS
Time series analysis
Error analysis
Statistical analysis
Vegetation
Sensors