|
ACCESS THE FULL ARTICLE
No SPIE Account? Create one
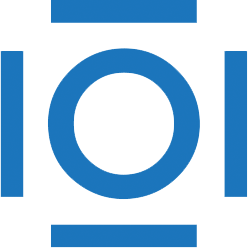
CITATIONS
Cited by 67 scholarly publications.
Machine learning
Pathology
Convolutional neural networks
Fine needle aspiration
Visualization
Cancer
Feature extraction