Purpose: Bayesian theory provides a sound framework for ultralow-dose computed tomography (ULdCT) image reconstruction with two terms for modeling the data statistical property and incorporating a priori knowledge for the image that is to be reconstructed. We investigate the feasibility of using a machine learning (ML) strategy, particularly the convolutional neural network (CNN), to construct a tissue-specific texture prior from previous full-dose computed tomography. Approach: Our study constructs four tissue-specific texture priors, corresponding with lung, bone, fat, and muscle, and integrates the prior with the prelog shift Poisson (SP) data property for Bayesian reconstruction of ULdCT images. The Bayesian reconstruction was implemented by an algorithm called SP-CNN-T and compared with our previous Markov random field (MRF)-based tissue-specific texture prior algorithm called SP-MRF-T. Results: In addition to conventional quantitative measures, mean squared error and peak signal-to-noise ratio, structure similarity index, feature similarity, and texture Haralick features were used to measure the performance difference between SP-CNN-T and SP-MRF-T algorithms in terms of the structure and tissue texture preservation, demonstrating the feasibility and the potential of the investigated ML approach. Conclusions: Both training performance and image reconstruction results showed the feasibility of constructing CNN texture prior model and the potential of improving the structure preservation of the nodule comparing to our previous regional tissue-specific MRF texture prior model. |
ACCESS THE FULL ARTICLE
No SPIE Account? Create one
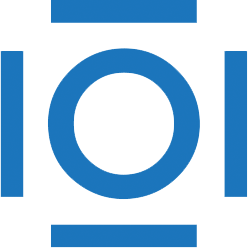
CITATIONS
Cited by 4 scholarly publications.
Tissues
Data modeling
Magnetorheological finishing
Machine learning
Image restoration
Reconstruction algorithms
Lung