|
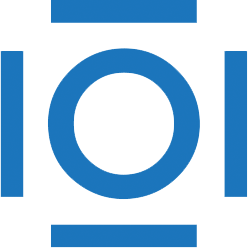
CITATIONS
Cited by 5 scholarly publications.
Hyperspectral imaging
Image classification
Algorithm development
Near infrared
Scene classification
Error analysis
Feature extraction