|
ACCESS THE FULL ARTICLE
No SPIE Account? Create one
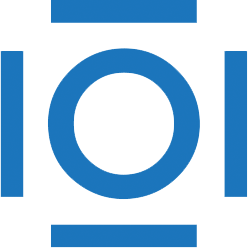
CITATIONS
Cited by 1 scholarly publication.
Lawrencium
LIDAR
Principal component analysis
Image segmentation
Feature selection
Image classification
Data modeling