|
ACCESS THE FULL ARTICLE
No SPIE Account? Create one
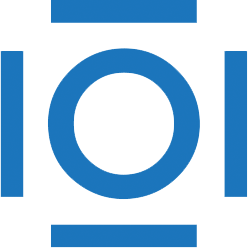
CITATIONS
Cited by 1 scholarly publication.
Distortion
Image quality
Convolutional neural networks
Computer programming
Databases
Associative arrays
Feature extraction