|
ACCESS THE FULL ARTICLE
No SPIE Account? Create one
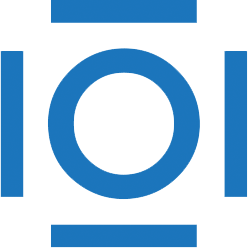
CITATIONS
Cited by 30 scholarly publications and 3 patents.
Magnetic resonance imaging
Signal to noise ratio
Fourier transforms
Ultrasonography
Motion models
Image restoration
Data modeling