Aircraft detection in remote-sensing images is a fundamental task in civil and military applications. Deep learning techniques to achieve end-to-end object detection have attracted the attention of the Earth observation community. One of the primary factors behind the success of deep learning techniques is the utilized data. Several previous studies focused on designing the network infrastructure. Instead, this study pays more attention to the data. With the increasing number of available public datasets, whether directly employing a large number of instances with great variation will lead to a good performance has become a research topic. The ways in which these object instances are collected differ greatly. For example, the image sizes, object sizes in the training images, and geospatial resolution are varied. Therefore, herein, the factors influencing the detection performance, such as the object size, ground sampling distance, and Google zoom view, are investigated. A you-only-look-once-v3-based detection process is proposed for automatic aircraft detection. A nonmaximum suppression algorithm strategy is applied to filter unreliable and redundant bounding boxes detected in the overlapping image blocks. The model generalization ability under different training data combinations is evaluated in several challenging cases. The results prove that more variety of training instances from a greater variety of zoom levels will result in more false alarms. Instead, more variety in the object sizes under a constant zoom level is welcome. A large range of aircraft sizes (i.e., 7 to 77 m in length in this study) can be detected, with a promising F1 score of 0.98. |
ACCESS THE FULL ARTICLE
No SPIE Account? Create one
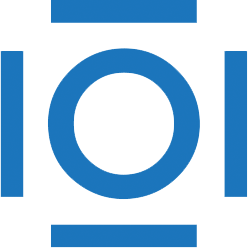
CITATIONS
Cited by 7 scholarly publications.
Data modeling
Satellites
Satellite imaging
Earth observing sensors
Zoom lenses
Performance modeling
Sensors