Hyperspectral imagery denoising is a classical problem in hyperspectral remote sensing applications. In our previous work, we combined principal component analysis with wavelet shrinkage, and good denoising results were obtained. We combine minimum noise fraction (MNF) with video block matching and 3D filtering (VBM3D), which is a powerful video denoising method. After MNF transform, we automatically select k0, the number of spectral band images as a threshold for denoising. We reduce the noise in spectral band images of MNF transformed data from k0 to the last band image and do not denoise the first k0 − 1 spectral band images. Finally, we perform an inverse MNF transform to obtain the denoised data cubes. We compare our MNF + VBM3D method with different denoising methods such as bivariate wavelet shrinkage (BivShrink), non-local means, SURELET, and block matching and 3D filtering (BM3D). Experimental results demonstrate that MNF + VBM3D achieves the best denoising results among almost all methods for three testing data cubes and with different noise levels. |
ACCESS THE FULL ARTICLE
No SPIE Account? Create one
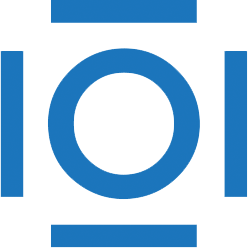
CITATIONS
Cited by 5 scholarly publications.
Denoising
Principal component analysis
Signal to noise ratio
Hyperspectral imaging
Video
3D image processing
Wavelets