Remote sensing is an effective way to analyze land surface changes on regular basis globally. In the previous literature, numerous change detection models were developed to detect the multitemporal or seasonal variations using different optical datasets, such as multispectral and hyperspectral. But there are many challenges involved in the processing of numerous bands, especially in the case of hyperspectral datasets, such as computational constraints and radiometric/atmospheric distortion. A simple framework-based artificial neural network (ANN) and post-classification comparison (PCC), named ANN-based PCC (ANPC), has been proposed to detect the multitemporal changes over agricultural land using hyperspectral, i.e., Earth Observation (EO-1) Hyperion and multispectral, i.e., Landsat-8. For cross-referencing, a comparative analysis is performed with respect to well-known PCC-based change detection methods, i.e., random forest-based post-comparison (RFPC) and support vector machine-based post-comparison (SVMPC). Experimental outcomes confirmed the effectiveness of ANPC (with an accuracy of more than 90%) in the extraction of multitemporal changes as compared to RFPC and SVMPC (with an accuracy of <90 % ). Our study enhances the utilization of the hyperspectral dataset (due to narrow spectral bands) in the extraction of critical information about the earth’s surface parameters. |
ACCESS THE FULL ARTICLE
No SPIE Account? Create one
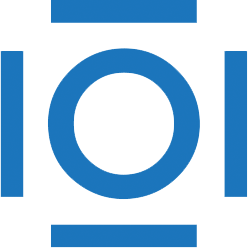
CITATIONS
Cited by 5 scholarly publications.
Earth observing sensors
Landsat
Artificial neural networks
Quantitative analysis
Accuracy assessment
Visualization
Data modeling