|
ACCESS THE FULL ARTICLE
No SPIE Account? Create one
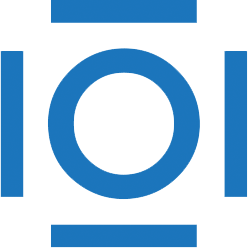
CITATIONS
Cited by 6 scholarly publications.
Laser phosphor displays
Optical engineering
Matrices
Spatial frequencies
Feature extraction
Image classification
Linear filtering