|
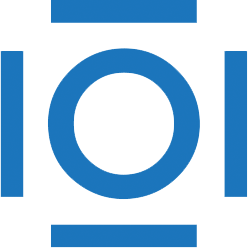
CITATIONS
Cited by 1 scholarly publication.
Hough transforms
Visualization
Image compression
Optical engineering
Image segmentation
Visual process modeling
Computer programming