A stable temperature calibration method based on radial basis function neural network (RBFNN) is proposed to obtain the complex relationship between the temperature and the center wavelength of fiber Bragg grating (FBG) with high accuracy and excellent stability. We introduce the regularized and generalized RBFNN, respectively, and test the accuracy of trained models. Experimental results demonstrate that the maximum absolute error (MAE) and root-mean-squared error (RMSE) of regularized RBFNN are 1.1098°C and 0.1982°C, respectively, in fitting and 1.0206°C and 0.1997°C, respectively, in testing, and the MAE and RMSE of generalized RBFNN are 1.1099°C and 0.1982°C, respectively, in fitting and 1.0209°C and 0.1997°C, respectively, in testing. Compared with existing methods, such as polynomial fitting and back propagation neural network, RBFNN has significantly improved the accuracy and stability of FBG temperature calibration and has considerable application prospect in FBG temperature measurement. |
ACCESS THE FULL ARTICLE
No SPIE Account? Create one
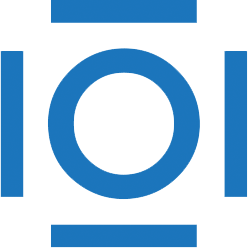
CITATIONS
Cited by 5 scholarly publications.
Fiber Bragg gratings
Neural networks
Temperature metrology
Calibration
Thermal effects
Optical engineering
Error analysis