Significance: We demonstrated the potential of using domain adaptation on functional near-infrared spectroscopy (fNIRS) data to classify different levels of n-back tasks that involve working memory. Aim: Domain shift in fNIRS data is a challenge in the workload level alignment across different experiment sessions and subjects. To address this problem, two domain adaptation approaches—Gromov–Wasserstein (G-W) and fused Gromov–Wasserstein (FG-W) were used. Approach: Specifically, we used labeled data from one session or one subject to classify trials in another session (within the same subject) or another subject. We applied G-W for session-by-session alignment and FG-W for subject-by-subject alignment to fNIRS data acquired during different n-back task levels. We compared these approaches with three supervised methods: multiclass support vector machine (SVM), convolutional neural network (CNN), and recurrent neural network (RNN). Results: In a sample of six subjects, G-W resulted in an alignment accuracy of 68 % ± 4 % (weighted mean ± standard error) for session-by-session alignment, FG-W resulted in an alignment accuracy of 55 % ± 2 % for subject-by-subject alignment. In each of these cases, 25% accuracy represents chance. Alignment accuracy results from both G-W and FG-W are significantly greater than those from SVM, CNN, and RNN. We also showed that removal of motion artifacts from the fNIRS data plays an important role in improving alignment performance. Conclusions: Domain adaptation has potential for session-by-session and subject-by-subject alignment of mental workload by using fNIRS data. |
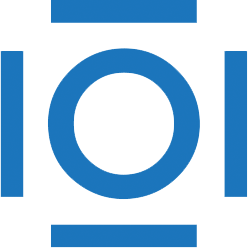
CITATIONS
Cited by 10 scholarly publications.
Matrices
Data modeling
Signal detection
Detection and tracking algorithms
Brain
Motion measurement
Sensors