ACCESS THE FULL ARTICLE
No SPIE Account? Create one
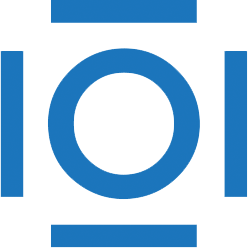
CITATIONS
Cited by 1 scholarly publication.
Optical proximity correction
Machine learning
Data modeling
Statistical modeling
Photomasks
Education and training
Performance modeling