|
ACCESS THE FULL ARTICLE
No SPIE Account? Create one
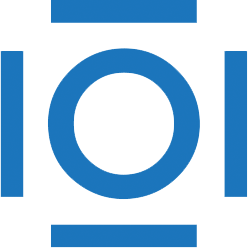
CITATIONS
Cited by 6 scholarly publications.
Transistors
Performance modeling
Semiconducting wafers
Monte Carlo methods
Instrument modeling
Distance measurement
Statistical modeling